Our research group Comprehensible Artificial Intelligence (CAI) has developed this demonstrator to show how innovative methods of Explainable AI can be used to improve the comprehensibility and trustworthiness of AI systems.
Using a use case from image-based quality control, we demonstrate how the use of machine learning in industry can be usefully extended by XAI methods to help companies achieve greater efficiency and higher product quality.
We invite you to explore our video podcast and actively participate in shaping the future of AI in industry.
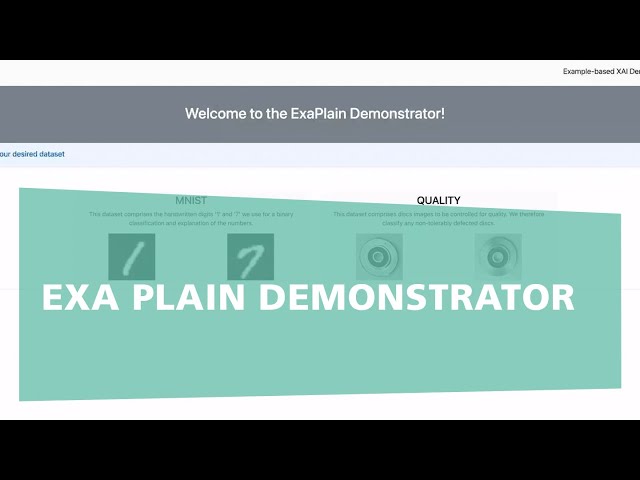