Forecasting demand for spare parts with supply chain analytics
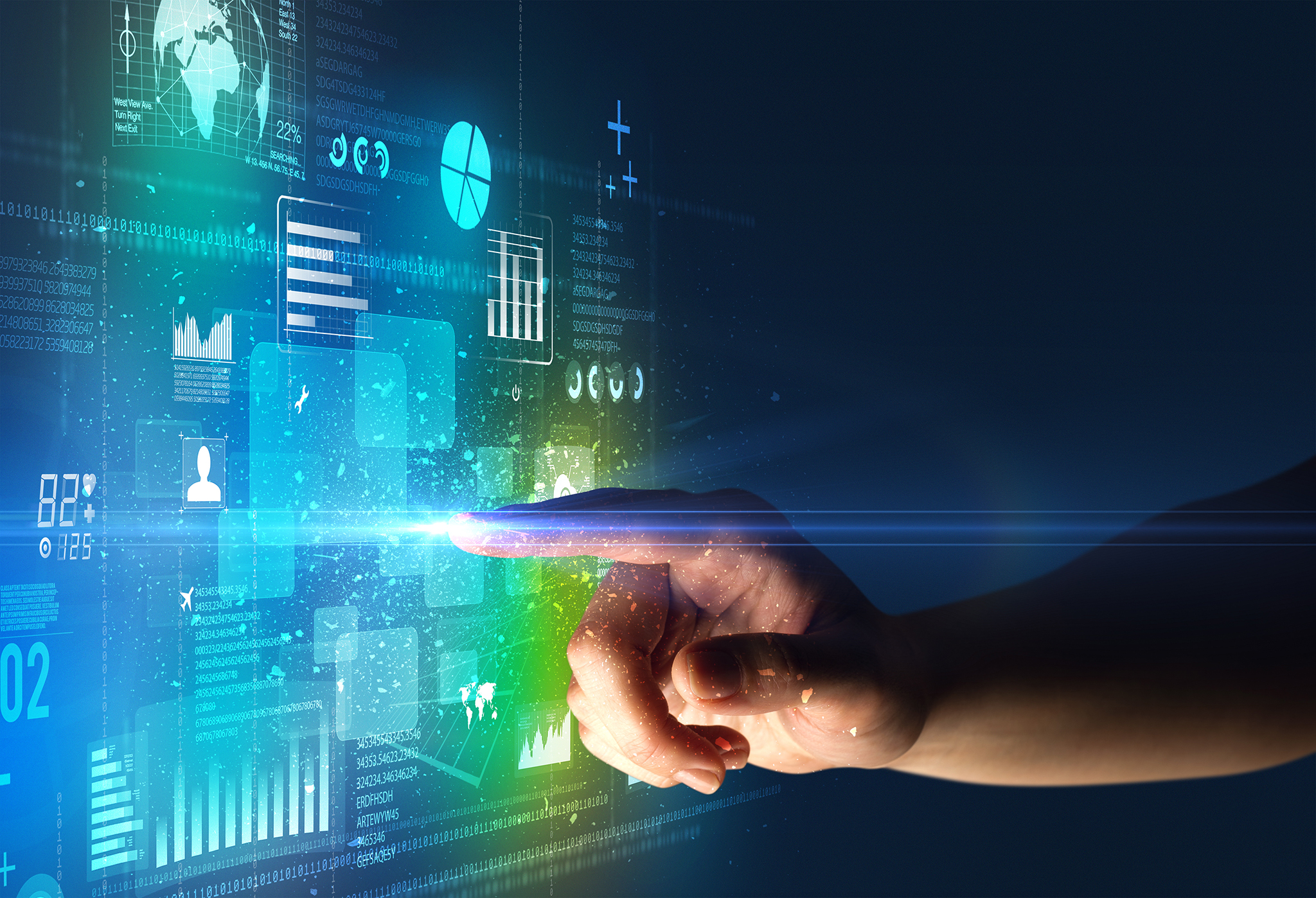
Data analytics offers a wide range of possibilities for optimizing applications in logistics. In addition to optimizing logistics networks or identifying KPIs, it holds huge potential for the prediction of critical events such as delays, customer demand and required stock levels. The question of which applications actually warrant the use of descriptive, predictive or prescriptive data analytics methods, and what data is needed in the first place, varies from case to case. The actual cost and benefit for companies becomes clear only during the concrete analytics use case.
For example, we worked with a manufacturer of domestic appliances to analyze its master and consumption data specifically for patterns and similarities. Using machine learning, a model was developed from this analysis for the long-term forecasting of the expected demand for spare parts. On this basis, the customer’s dispatchers can now plan and utilize their stock levels in an optimal manner.
We have built up a library with analytics use cases and linked them with useful context such as information about the corresponding business processes and the data required for them. This enables companies to quickly find applications that are interesting for them, transfer these applications to their own business processes and evaluate them, and initialize analytics projects.