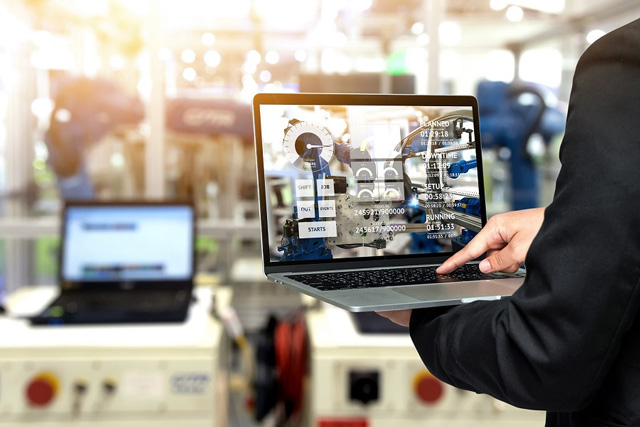
Automated machine learning (AutoML) for industrial applications
There is a big obstacle when using machine learning (ML) for practical applications: identifying the best methods and their ideal configuration – in other words, adapting the existing data situation, processes and application requirements – is extremely complicated and expensive. AutoML promises to remedy this. It harnesses systems that automate the development and configuration of AI processes. Up to now, existing AutoML systems have generally been used in scientific and research contexts because these are geared toward a few standard ML processes and optimized data situations. By way of contrast, it is rare to find such optimum conditions in industrial practice, which explains why limited use has been made of AutoML here to date. With our project, we set out to research how suitable AutoML solutions are for practical use and how they can be transferred to complex industrial applications.
Together with an industry client, we investigated if AutoML systems make sense in an industrial context, if standard systems are sufficient and which specific adjustments would be required to make it worth rolling these systems out. To do so, we performed precision analysis of the state of research, tested the limits and requirements of existing systems and developed our own AutoML system for quality control. We found that specifically configured AutoML systems generally lead to an increase in both performance and output. For this reason, targeted AutoML solutions for special applications are a promising research area for the future.